Human Performance Modeling
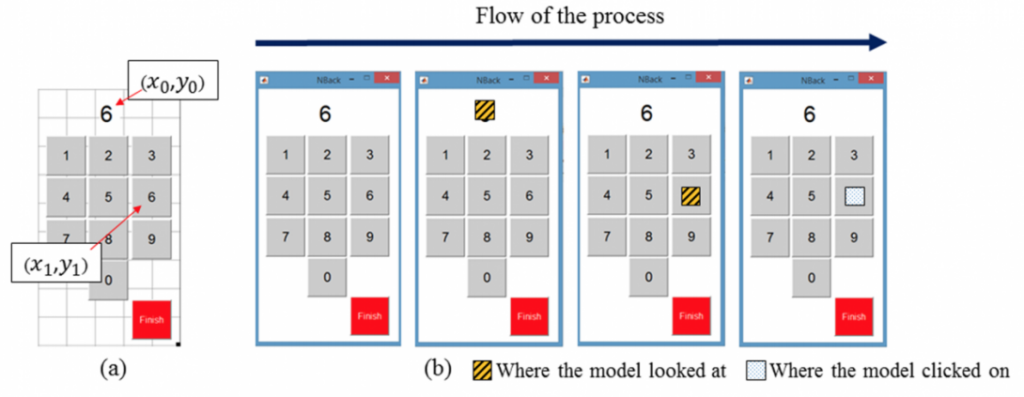
Modeling of Stimulus-Response Secondary Tasks with Different Modalities While Driving in a Computational Cognitive Architecture. In Proceedings of the 9th International Driving Symposium on Human Factors in Driver Assessment, Training, and Vehicle Design. DOI: 10.17077/drivingassessment.1615.
This is a computational human performance modeling study based upon the queueing network cognitive architecture to predict driver’s eye glances and workload for four stimulus-response secondary tasks (i.e., auditory-manual, auditory-speech, visual-manual, and visual-speech types) while driving. The model was evaluated with the empirical data from 24 subjects, and the percentage of eyes-off-road time and driver workload generated by the model were similar to the human subject data. Future studies aim to extend the types of voice announcements/commands to enable Human-Machine-Interface (HMI) evaluations with a wider range of usability test for in-vehicle infotainment system developments.
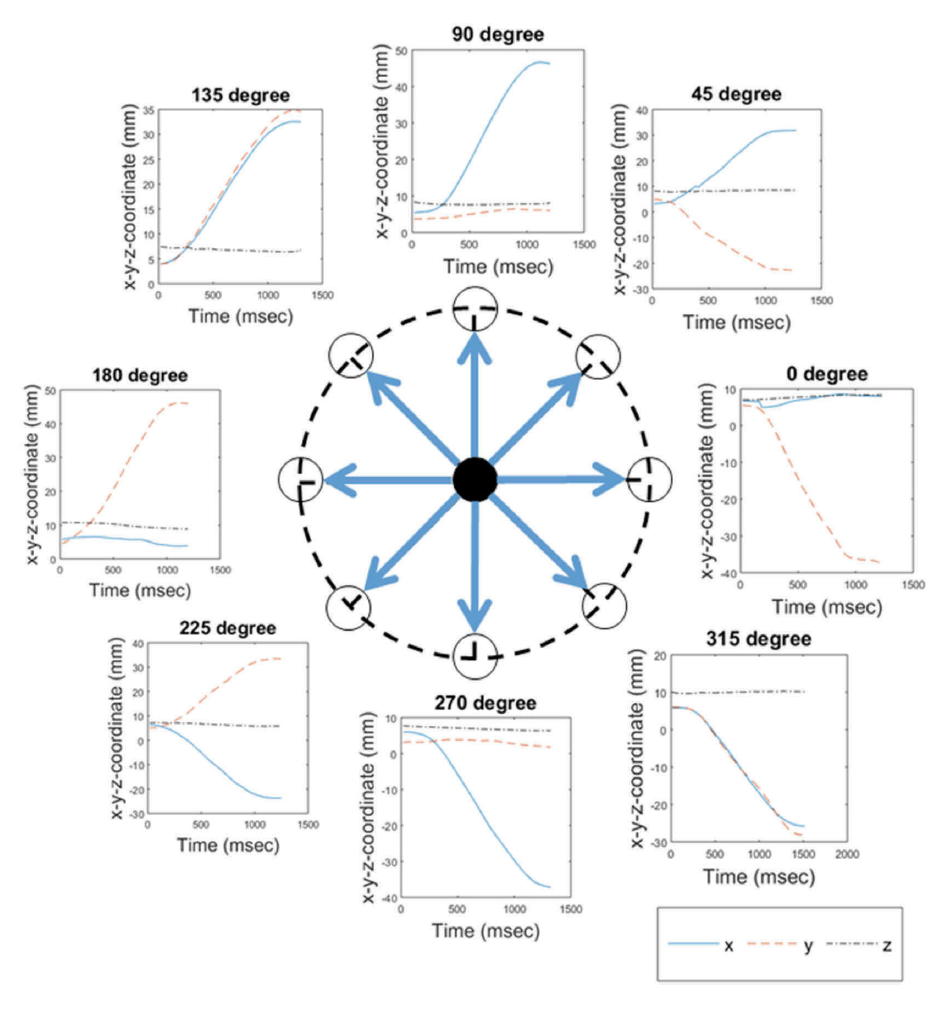
Computational modeling of touchscreen drag gestures using a cognitive architecture and motion tracking. International Journal of Human-Computer Interaction. DOI: 10.1080/10447318.2018.1466858.
This article presents a computational model that predicts finger-drag gesture performance on touchscreen devices, by integrating the queueing network (QN) cognitive architecture and motion tracking. Specifically, the QN-based model was developed to predict two execution times: the finger movement time of drag-gesture (i.e., only the motion time of the finger touched and dragged on the surface of touchscreen) and the comprehensive process time of drag-gesture (i.e., the entire process time to complete the finger-drag task, including visual attention shift, memory storage and retrieval, and hand-finger movements). To develop predictive models for the finger movement time of drag-gesture, 11 participants’ motion data were collected and a regression analysis with parameters of hand-finger anthropometric data and eight angular directions was conducted. Human subject data from our previous study (Jeong & Liu, 2017a) were used to evaluate the QN-based model, generating similar outputs (R2 was more than 80% and root-mean square was less than 300 msec) for both execution times.
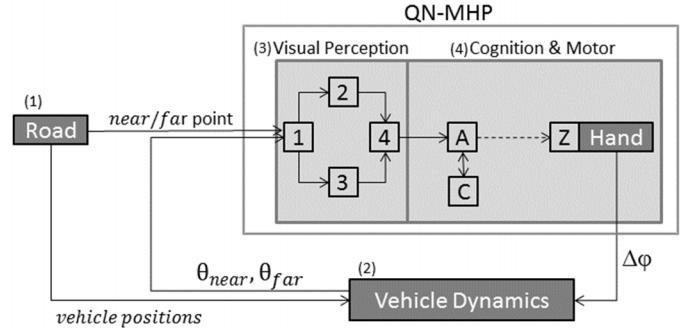
Computational Modeling of Driver Lateral Control on Curved Roads with Integration of Vehicle Dynamics and Reference Trajectory Tracking. In Proceedings of the 9th International Driving Symposium on Human Factors in Driver Assessment, Training, and Vehicle Design. DOI: 10.17077/drivingassessment.1635.
Driver’s lateral control on curved roads plays a significant role in reducing or avoiding the crashes. To understand and predict driver performance on curved roads, a computational model was developed in a cognitive architecture, the Queueing Network-Model Human Processor (QN-MHP), with the integration of vehicle dynamics principles (i.e., how to steer based on near and far angles) and the reference trajectory tracking method (i.e., how to steer on the road varying with radius of road curvature). The model was implemented with four major components: road information, vehicle dynamics, visual perception, and cognition & motor controls. The model outputs were validated with the corresponding human subject performance in the literature. The performance results of the model highly fitted the human subject data such as steering wheel angle.